Dutch Rail employs predictive maintenance
28/02/2019
Engineers in the Netherlands have improved rail reliability by taking a new predictive approach to track maintenance.In 2009, rail maintenance in the Netherlands took its first steps towards a radical transformation when it moved from being a descriptive to a predictive maintenance regime.
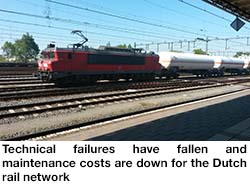
The company behind the move is Asset Rail, a joint venture between Arcadis and Dutch contractor Dura Vermeer.
Initially the company had only one contract, but the approach has been so successful that it now has five and maintains 25% of the 7000 km of track on the Dutch network. Technical failures have fallen by 64% and maintenance costs are down by 40%.
Previously, repairs were carried out according to a standard, with no real connection between the cause of a failure and the reason to maintain a piece of infrastructure. If defects were spotted, contractors had no incentive to carry out repairs immediately as it was more lucrative for them to complete the work during overtime hours.
Delays, failure rates and costs mounted and passengers became increasingly frustrated. However, under the new system contractors are incentivised to deliver an agreed level of performance.
At the heart of the new thinking is the joint venture’s failure mode, effects and criticality analysis (FMECA).
Using targeted data analytics combined with technical insights, Arcadis and Dura Vermeer were able to start thinking about the failure mode and the maintenance strategies so that problems could be identified at an optimal point before failure. Contractors would then be able to pre-emptively carry out repairs, reducing delays.
The first step in the process was for the team to build up a good understanding of the asset base and the range of components, switches and other asset sets. The data was then collected. The team used measurement trains, remote condition monitoring, sensors on drones and other data input sources to build up a picture of a component’s performance.
By connecting the different sets of data and using quantitative modelling, the team was able to find the underlying relationships between and dependencies of the assets, including their use and performance. Before these methods were introduced, the network had many different smart assets, but little was being done with the data collected from them.